AI and Data Aggregation
“Without data, you're just another person with an opinion.” – W. Edwards Deming
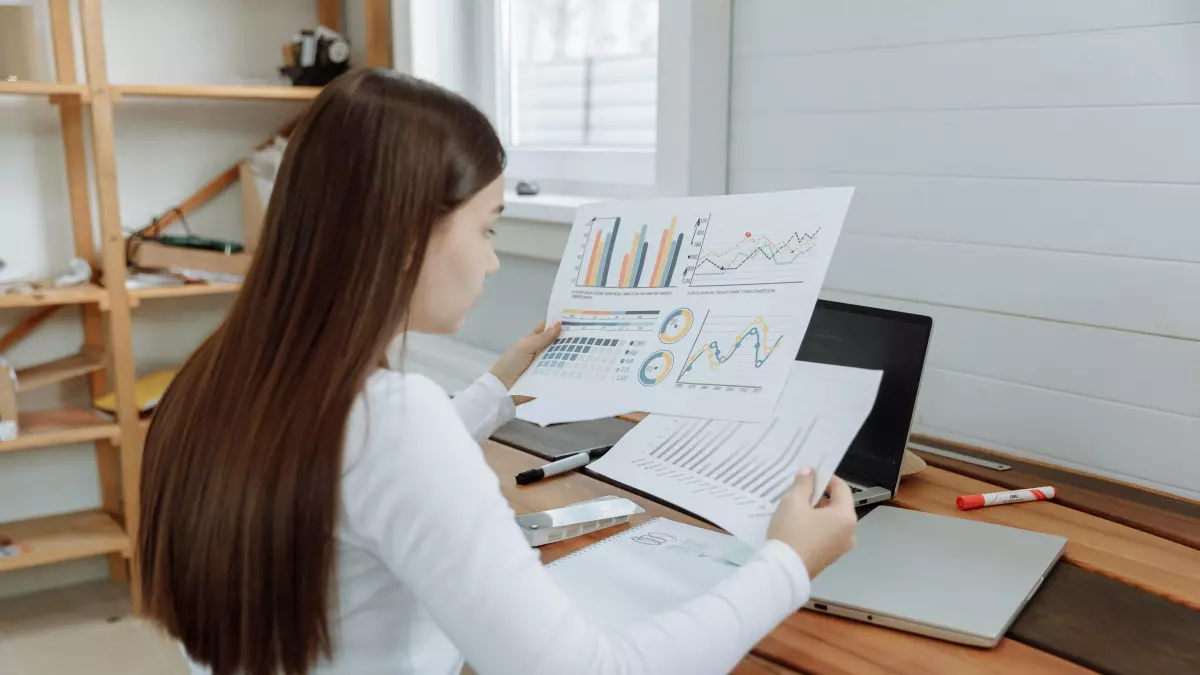
By Tomás Oliveira
Deming's words ring truer than ever in the age of AI and big data. But here’s the thing: having data isn’t enough. You need to make sense of it, and that’s where data aggregation comes in. It’s the process of collecting and summarizing data from multiple sources to provide a unified view. Sounds simple, right? Well, not when you’re dealing with terabytes of information coming from all directions. This is where AI steps in, making data aggregation faster, smarter, and more efficient.
Why Data Aggregation Matters
Imagine you’re running a business with data coming from social media, sales platforms, customer service, and more. Each data point tells a part of the story, but without aggregating it, you’re left with a fragmented narrative. Data aggregation helps you pull all these pieces together, giving you a complete picture of what’s really going on. It’s like assembling a puzzle—except the puzzle has millions of pieces, and they keep changing.
Traditionally, data aggregation was a manual, time-consuming process. Analysts would spend hours or even days pulling data from different sources, cleaning it, and then summarizing it. But with AI, this process can be automated, allowing businesses to get real-time insights without the headache.
How AI Transforms Data Aggregation
AI doesn’t just speed up data aggregation—it makes it smarter. Here’s how:
- Automated Data Collection: AI can automatically pull data from multiple sources, whether it’s structured data from databases or unstructured data from social media, emails, or even IoT devices. No more manual data scraping!
- Data Cleaning: AI algorithms can detect and fix inconsistencies in the data, ensuring that the aggregated data is accurate and reliable. This is crucial because bad data leads to bad decisions.
- Real-Time Processing: With AI, data aggregation can happen in real-time, allowing businesses to make decisions based on the most up-to-date information. This is a game-changer for industries like finance or e-commerce, where timing is everything.
- Pattern Recognition: AI can identify patterns and correlations in the aggregated data that humans might miss. This can lead to new insights and opportunities that would have otherwise gone unnoticed.
AI-Powered Aggregation in Action
Let’s look at a few examples of how AI-powered data aggregation is being used in the real world:
- Healthcare: Hospitals and clinics are using AI to aggregate patient data from various sources—medical records, lab results, wearable devices—to provide doctors with a comprehensive view of a patient’s health. This allows for more personalized and effective treatment plans.
- Finance: Financial institutions are using AI to aggregate data from market trends, customer transactions, and economic reports. This helps them make better investment decisions and manage risks more effectively.
- Retail: Retailers are using AI to aggregate data from sales, customer feedback, and social media to understand consumer behavior and predict future trends. This allows them to optimize inventory, pricing, and marketing strategies.
Challenges of AI in Data Aggregation
Of course, AI isn’t a magic bullet. There are still challenges when it comes to AI-powered data aggregation:
- Data Privacy: Aggregating data from multiple sources can raise privacy concerns, especially when dealing with sensitive information. Companies need to ensure they comply with data protection regulations like GDPR.
- Bias in Data: AI algorithms are only as good as the data they’re trained on. If the data is biased, the aggregated insights will be too. It’s important to regularly audit AI systems to ensure they’re providing accurate and unbiased results.
- Cost: Implementing AI-powered data aggregation systems can be expensive, especially for smaller businesses. However, the long-term benefits often outweigh the initial investment.
The Future of AI in Data Aggregation
As AI continues to evolve, we can expect even more advanced data aggregation techniques. For example, AI could start to predict future trends based on aggregated data, allowing businesses to make proactive decisions rather than reactive ones. We might also see more integration of AI with blockchain technology, ensuring that aggregated data is not only accurate but also secure and tamper-proof.
So, what’s the takeaway here? AI is revolutionizing data aggregation, making it faster, smarter, and more efficient. But like any tool, it’s not perfect. Businesses need to be aware of the challenges and take steps to mitigate them. Still, if you’re not using AI for data aggregation yet, you’re missing out on a powerful way to unlock deeper insights from your data.
Ready to take the plunge into AI-powered data aggregation? It’s time to stop drowning in data and start making sense of it.